PyTorch tutorial¶
Data collection¶
In this PyTorch tutorial, we use GTZAN dataset which consists of 10 exclusive genre classes. Please run the following script in your local path.
!wget http://opihi.cs.uvic.ca/sound/genres.tar.gz
!tar -zxvf genres.tar.gz
!wget https://raw.githubusercontent.com/coreyker/dnn-mgr/master/gtzan/train_filtered.txt
!wget https://raw.githubusercontent.com/coreyker/dnn-mgr/master/gtzan/valid_filtered.txt
!wget https://raw.githubusercontent.com/coreyker/dnn-mgr/master/gtzan/test_filtered.txt
Data loader¶
import os
import random
import torch
import numpy as np
import soundfile as sf
from torch.utils import data
from torchaudio_augmentations import (
RandomResizedCrop,
RandomApply,
PolarityInversion,
Noise,
Gain,
HighLowPass,
Delay,
PitchShift,
Reverb,
Compose,
)
GTZAN_GENRES = ['blues', 'classical', 'country', 'disco', 'hiphop', 'jazz', 'metal', 'pop', 'reggae', 'rock']
class GTZANDataset(data.Dataset):
def __init__(self, data_path, split, num_samples, num_chunks, is_augmentation):
self.data_path = data_path if data_path else ''
self.split = split
self.num_samples = num_samples
self.num_chunks = num_chunks
self.is_augmentation = is_augmentation
self.genres = GTZAN_GENRES
self._get_song_list()
if is_augmentation:
self._get_augmentations()
def _get_song_list(self):
list_filename = os.path.join(self.data_path, '%s_filtered.txt' % self.split)
with open(list_filename) as f:
lines = f.readlines()
self.song_list = [line.strip() for line in lines]
def _get_augmentations(self):
transforms = [
RandomResizedCrop(n_samples=self.num_samples),
RandomApply([PolarityInversion()], p=0.8),
RandomApply([Noise(min_snr=0.3, max_snr=0.5)], p=0.3),
RandomApply([Gain()], p=0.2),
RandomApply([HighLowPass(sample_rate=22050)], p=0.8),
RandomApply([Delay(sample_rate=22050)], p=0.5),
RandomApply([PitchShift(n_samples=self.num_samples, sample_rate=22050)], p=0.4),
RandomApply([Reverb(sample_rate=22050)], p=0.3),
]
self.augmentation = Compose(transforms=transforms)
def _adjust_audio_length(self, wav):
if self.split == 'train':
random_index = random.randint(0, len(wav) - self.num_samples - 1)
wav = wav[random_index : random_index + self.num_samples]
else:
hop = (len(wav) - self.num_samples) // self.num_chunks
wav = np.array([wav[i * hop : i * hop + self.num_samples] for i in range(self.num_chunks)])
return wav
def __getitem__(self, index):
line = self.song_list[index]
# get genre
genre_name = line.split('/')[0]
genre_index = self.genres.index(genre_name)
# get audio
audio_filename = os.path.join(self.data_path, 'genres', line)
wav, fs = sf.read(audio_filename)
# adjust audio length
wav = self._adjust_audio_length(wav).astype('float32')
# data augmentation
if self.is_augmentation:
wav = self.augmentation(torch.from_numpy(wav).unsqueeze(0)).squeeze(0).numpy()
return wav, genre_index
def __len__(self):
return len(self.song_list)
def get_dataloader(data_path=None,
split='train',
num_samples=22050 * 29,
num_chunks=1,
batch_size=16,
num_workers=0,
is_augmentation=False):
is_shuffle = True if (split == 'train') else False
batch_size = batch_size if (split == 'train') else (batch_size // num_chunks)
data_loader = data.DataLoader(dataset=GTZANDataset(data_path,
split,
num_samples,
num_chunks,
is_augmentation),
batch_size=batch_size,
shuffle=is_shuffle,
drop_last=False,
num_workers=num_workers)
return data_loader
Let’s check returned data shapes.
train_loader = get_dataloader(split='train', is_augmentation=True)
iter_train_loader = iter(train_loader)
train_wav, train_genre = next(iter_train_loader)
valid_loader = get_dataloader(split='valid')
test_loader = get_dataloader(split='test')
iter_test_loader = iter(test_loader)
test_wav, test_genre = next(iter_test_loader)
print('training data shape: %s' % str(train_wav.shape))
print('validation/test data shape: %s' % str(test_wav.shape))
print(train_genre)
training data shape: torch.Size([16, 639450])
validation/test data shape: torch.Size([16, 1, 639450])
tensor([9, 3, 4, 2, 2, 5, 2, 5, 7, 1, 1, 7, 8, 7, 4, 0])
Note
A data loader returns a tensor of audio and their genre indice at each iteration.
Random chunks of audio are cropped from the entire sequence during the training. But in validation / test phase, an entire sequence is split into multiple chunks and the chunks are stacked. The stacked chunks are later input to a trained model and the output predictions are aggregated to make song-level predictions.
Model¶
We are going to build a simple 2D CNN model with Mel spectrogram inputs. First, we design a convolution module that consists of 3x3 convolution, batch normalization, ReLU non-linearity, and 2x2 max pooling. This module is going to be used for each layer of the 2D CNN.
from torch import nn
class Conv_2d(nn.Module):
def __init__(self, input_channels, output_channels, shape=3, pooling=2, dropout=0.1):
super(Conv_2d, self).__init__()
self.conv = nn.Conv2d(input_channels, output_channels, shape, padding=shape//2)
self.bn = nn.BatchNorm2d(output_channels)
self.relu = nn.ReLU()
self.maxpool = nn.MaxPool2d(pooling)
self.dropout = nn.Dropout(dropout)
def forward(self, wav):
out = self.conv(wav)
out = self.bn(out)
out = self.relu(out)
out = self.maxpool(out)
out = self.dropout(out)
return out
Stack the convolution layers. In a PyTorch module, layers are declared in __init__
and they are built up in forward
function.
import torchaudio
class CNN(nn.Module):
def __init__(self, num_channels=16,
sample_rate=22050,
n_fft=1024,
f_min=0.0,
f_max=11025.0,
num_mels=128,
num_classes=10):
super(CNN, self).__init__()
# mel spectrogram
self.melspec = torchaudio.transforms.MelSpectrogram(sample_rate=sample_rate,
n_fft=n_fft,
f_min=f_min,
f_max=f_max,
n_mels=num_mels)
self.amplitude_to_db = torchaudio.transforms.AmplitudeToDB()
self.input_bn = nn.BatchNorm2d(1)
# convolutional layers
self.layer1 = Conv_2d(1, num_channels, pooling=(2, 3))
self.layer2 = Conv_2d(num_channels, num_channels, pooling=(3, 4))
self.layer3 = Conv_2d(num_channels, num_channels * 2, pooling=(2, 5))
self.layer4 = Conv_2d(num_channels * 2, num_channels * 2, pooling=(3, 3))
self.layer5 = Conv_2d(num_channels * 2, num_channels * 4, pooling=(3, 4))
# dense layers
self.dense1 = nn.Linear(num_channels * 4, num_channels * 4)
self.dense_bn = nn.BatchNorm1d(num_channels * 4)
self.dense2 = nn.Linear(num_channels * 4, num_classes)
self.dropout = nn.Dropout(0.5)
self.relu = nn.ReLU()
def forward(self, wav):
# input Preprocessing
out = self.melspec(wav)
out = self.amplitude_to_db(out)
# input batch normalization
out = out.unsqueeze(1)
out = self.input_bn(out)
# convolutional layers
out = self.layer1(out)
out = self.layer2(out)
out = self.layer3(out)
out = self.layer4(out)
out = self.layer5(out)
# reshape. (batch_size, num_channels, 1, 1) -> (batch_size, num_channels)
out = out.reshape(len(out), -1)
# dense layers
out = self.dense1(out)
out = self.dense_bn(out)
out = self.relu(out)
out = self.dropout(out)
out = self.dense2(out)
return out
Note
In this example, we performed preprocessing on-the-fly using torchaudio. This process can be done offline outside of the network using other libraries such as librosa and essentia.
Tip
There is no activation function at the last layer since
nn.CrossEntropyLoss
already includes softmax in it.If you want to perform multi-label binary classification, include
out = nn.Sigmoid()(out)
at the last layer and usenn.BCELoss()
.
Training¶
Iterate training. One epoch is defined as visiting all training items once. This definition can be modified in def __len__
in data loader.
from sklearn.metrics import accuracy_score, confusion_matrix
device = torch.device('cuda:0' if torch.cuda.is_available() else 'cpu')
cnn = CNN().to(device)
loss_function = nn.CrossEntropyLoss()
optimizer = torch.optim.Adam(cnn.parameters(), lr=0.001)
valid_losses = []
num_epochs = 30
for epoch in range(num_epochs):
losses = []
# Train
cnn.train()
for (wav, genre_index) in train_loader:
wav = wav.to(device)
genre_index = genre_index.to(device)
# Forward
out = cnn(wav)
loss = loss_function(out, genre_index)
# Backward
optimizer.zero_grad()
loss.backward()
optimizer.step()
losses.append(loss.item())
print('Epoch: [%d/%d], Train loss: %.4f' % (epoch+1, num_epochs, np.mean(losses)))
# Validation
cnn.eval()
y_true = []
y_pred = []
losses = []
for wav, genre_index in valid_loader:
wav = wav.to(device)
genre_index = genre_index.to(device)
# reshape and aggregate chunk-level predictions
b, c, t = wav.size()
logits = cnn(wav.view(-1, t))
logits = logits.view(b, c, -1).mean(dim=1)
loss = loss_function(logits, genre_index)
losses.append(loss.item())
_, pred = torch.max(logits.data, 1)
# append labels and predictions
y_true.extend(genre_index.tolist())
y_pred.extend(pred.tolist())
accuracy = accuracy_score(y_true, y_pred)
valid_loss = np.mean(losses)
print('Epoch: [%d/%d], Valid loss: %.4f, Valid accuracy: %.4f' % (epoch+1, num_epochs, valid_loss, accuracy))
# Save model
valid_losses.append(valid_loss.item())
if np.argmin(valid_losses) == epoch:
print('Saving the best model at %d epochs!' % epoch)
torch.save(cnn.state_dict(), 'best_model.ckpt')
Epoch: [1/30], Train loss: 2.4078
Epoch: [1/30], Valid loss: 2.3558, Valid accuracy: 0.1117
Saving the best model at 0 epochs!
Epoch: [2/30], Train loss: 2.3422
Epoch: [2/30], Valid loss: 2.2748, Valid accuracy: 0.1218
Saving the best model at 1 epochs!
Epoch: [3/30], Train loss: 2.2830
Epoch: [3/30], Valid loss: 2.2013, Valid accuracy: 0.1929
Saving the best model at 2 epochs!
Epoch: [4/30], Train loss: 2.2026
Epoch: [4/30], Valid loss: 2.0716, Valid accuracy: 0.2487
Saving the best model at 3 epochs!
Epoch: [5/30], Train loss: 2.1279
Epoch: [5/30], Valid loss: 1.9948, Valid accuracy: 0.2640
Saving the best model at 4 epochs!
Epoch: [6/30], Train loss: 2.1007
Epoch: [6/30], Valid loss: 1.9407, Valid accuracy: 0.3249
Saving the best model at 5 epochs!
Epoch: [7/30], Train loss: 2.0670
Epoch: [7/30], Valid loss: 1.9217, Valid accuracy: 0.3096
Saving the best model at 6 epochs!
Epoch: [8/30], Train loss: 2.0387
Epoch: [8/30], Valid loss: 1.9618, Valid accuracy: 0.2893
Epoch: [9/30], Train loss: 2.0034
Epoch: [9/30], Valid loss: 1.7882, Valid accuracy: 0.3604
Saving the best model at 8 epochs!
Epoch: [10/30], Train loss: 1.9669
Epoch: [10/30], Valid loss: 1.7608, Valid accuracy: 0.3807
Saving the best model at 9 epochs!
Epoch: [11/30], Train loss: 1.9212
Epoch: [11/30], Valid loss: 1.7428, Valid accuracy: 0.3604
Saving the best model at 10 epochs!
Epoch: [12/30], Train loss: 1.9497
Epoch: [12/30], Valid loss: 1.7381, Valid accuracy: 0.3401
Saving the best model at 11 epochs!
Epoch: [13/30], Train loss: 1.8578
Epoch: [13/30], Valid loss: 1.7946, Valid accuracy: 0.3350
Epoch: [14/30], Train loss: 1.8934
Epoch: [14/30], Valid loss: 1.6822, Valid accuracy: 0.3959
Saving the best model at 13 epochs!
Epoch: [15/30], Train loss: 1.8459
Epoch: [15/30], Valid loss: 1.6475, Valid accuracy: 0.4416
Saving the best model at 14 epochs!
Epoch: [16/30], Train loss: 1.8433
Epoch: [16/30], Valid loss: 1.6429, Valid accuracy: 0.3503
Saving the best model at 15 epochs!
Epoch: [17/30], Train loss: 1.8358
Epoch: [17/30], Valid loss: 2.0232, Valid accuracy: 0.3046
Epoch: [18/30], Train loss: 1.8106
Epoch: [18/30], Valid loss: 1.6712, Valid accuracy: 0.3655
Epoch: [19/30], Train loss: 1.7393
Epoch: [19/30], Valid loss: 2.2497, Valid accuracy: 0.2741
Epoch: [20/30], Train loss: 1.7158
Epoch: [20/30], Valid loss: 1.5637, Valid accuracy: 0.4162
Saving the best model at 19 epochs!
Epoch: [21/30], Train loss: 1.7603
Epoch: [21/30], Valid loss: 1.4845, Valid accuracy: 0.5178
Saving the best model at 20 epochs!
Epoch: [22/30], Train loss: 1.7305
Epoch: [22/30], Valid loss: 1.6282, Valid accuracy: 0.3503
Epoch: [23/30], Train loss: 1.7213
Epoch: [23/30], Valid loss: 1.4270, Valid accuracy: 0.5381
Saving the best model at 22 epochs!
Epoch: [24/30], Train loss: 1.7064
Epoch: [24/30], Valid loss: 1.6344, Valid accuracy: 0.3655
Epoch: [25/30], Train loss: 1.6306
Epoch: [25/30], Valid loss: 1.3873, Valid accuracy: 0.5330
Saving the best model at 24 epochs!
Epoch: [26/30], Train loss: 1.7458
Epoch: [26/30], Valid loss: 1.4194, Valid accuracy: 0.5076
Epoch: [27/30], Train loss: 1.6578
Epoch: [27/30], Valid loss: 1.7264, Valid accuracy: 0.3604
Epoch: [28/30], Train loss: 1.6247
Epoch: [28/30], Valid loss: 1.4872, Valid accuracy: 0.5076
Epoch: [29/30], Train loss: 1.6642
Epoch: [29/30], Valid loss: 1.3975, Valid accuracy: 0.4772
Epoch: [30/30], Train loss: 1.6681
Epoch: [30/30], Valid loss: 1.6023, Valid accuracy: 0.4213
Evaluation¶
Collect the trained model’s predictions for the test set. Chunk-level predictions are aggregated to make song-level predictions.
# Load the best model
S = torch.load('best_model.ckpt')
cnn.load_state_dict(S)
print('loaded!')
# Run evaluation
cnn.eval()
y_true = []
y_pred = []
with torch.no_grad():
for wav, genre_index in test_loader:
wav = wav.to(device)
genre_index = genre_index.to(device)
# reshape and aggregate chunk-level predictions
b, c, t = wav.size()
logits = cnn(wav.view(-1, t))
logits = logits.view(b, c, -1).mean(dim=1)
_, pred = torch.max(logits.data, 1)
# append labels and predictions
y_true.extend(genre_index.tolist())
y_pred.extend(pred.tolist())
loaded!
Finally, we can assess the performance and visualize a confusion matrix for better understanding.
import seaborn as sns
from sklearn.metrics import confusion_matrix
accuracy = accuracy_score(y_true, y_pred)
cm = confusion_matrix(y_true, y_pred)
sns.heatmap(cm, annot=True, xticklabels=GTZAN_GENRES, yticklabels=GTZAN_GENRES, cmap='YlGnBu')
print('Accuracy: %.4f' % accuracy)
Accuracy: 0.5414
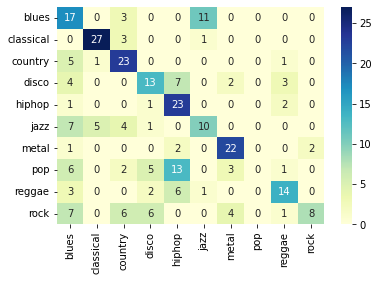
Tip
In this tutorial, we did not use any high-level library for more understandable implementation. We highly recommend checking the following libraries for simplified implementation: